The Essential Guide to Data Labeling for Businesses
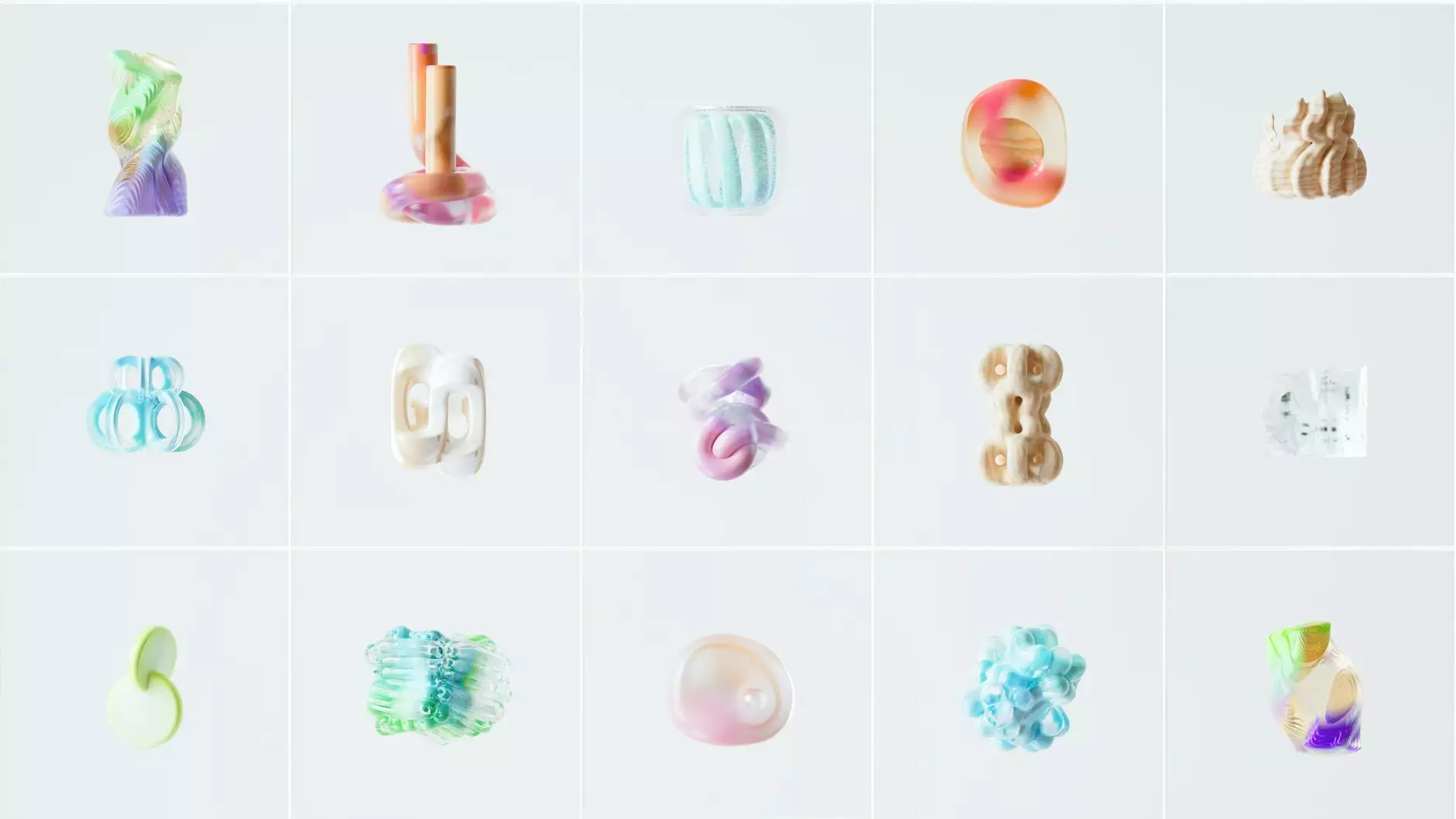
In the rapidly evolving landscape of artificial intelligence and machine learning, the concept of data labeling has emerged as a cornerstone of successful projects. As more businesses recognize the potential of AI, they are realizing that the quality of their data directly influences the outcomes of their algorithms. With platforms like Keylabs.ai offering robust data annotation tools and comprehensive data annotation platforms, understanding the significance of data labeling is crucial for any organization aiming to leverage AI technologies effectively.
What is Data Labeling?
Data labeling is the process of annotating or tagging data with informative labels to train machine learning models. This is vital for supervised learning, where algorithms learn from a set of input-output pairs.
The Process of Data Labeling
Data labeling involves several key steps:
- Data Collection: Gathering raw data from various sources.
- Data Preparation: Cleaning and organizing the data to make it suitable for annotation.
- Annotation: Marking data with labels that provide context (e.g., identifying objects in images).
- Quality Assurance: Verifying the accuracy of the labeled data.
- Utilization: Feeding the labeled data into machine learning algorithms for training.
Importance of Data Labeling in AI
The efficacy of AI models heavily relies on the quality of the training data. Here’s why data labeling is crucial:
- Enhanced Accuracy: Properly labeled datasets lead to more accurate predictions by the model.
- Improved Learning: Machine learning algorithms require high-quality labeled data to identify patterns effectively.
- Faster Development: Well-structured annotated datasets accelerate the model training process, reducing time-to-market.
- Scalability: Efficient data labeling processes allow businesses to scale their AI initiatives rapidly.
Challenges in Data Labeling
Despite its significance, there are challenges involved in the data labeling process:
1. Volume of Data
As the volume of data generated by businesses increases exponentially, managing, annotating, and maintaining quality becomes increasingly challenging.
2. Complexity of Tasks
Labeling complex data types such as images, videos, and audio can be labor-intensive and requires specialized knowledge.
3. Ensuring Quality
Maintaining consistency and accuracy in labeling is critical but often difficult, especially when done manually.
How Keylabs.ai Can Transform Your Data Annotation Efforts
When it comes to overcoming the challenges of data labeling, Keylabs.ai stands out as a data annotation platform designed to streamline the entire process. Here’s how:
1. Advanced Annotation Tools
Keylabs.ai offers intuitive and powerful annotation tools that accommodate various data types, ensuring that team members can accurately label data without significant training.
2. Automation and Efficiency
The platform harnesses the power of AI to assist in automating routine tagging tasks, reducing human workload and speeding up the annotation process.
3. Collaborative Environment
Your teams can work together in real-time, promoting collaboration and ensuring that everyone is on the same page — an essential part of maintaining quality in data labeling.
4. Quality Assurance Protocols
Keylabs.ai incorporates built-in quality checks and balances to ensure that your labeled data meets the highest standards. This minimizes errors and enhances the end product's reliability.