Machine Learning in Malware Detection: Revolutionizing Cybersecurity
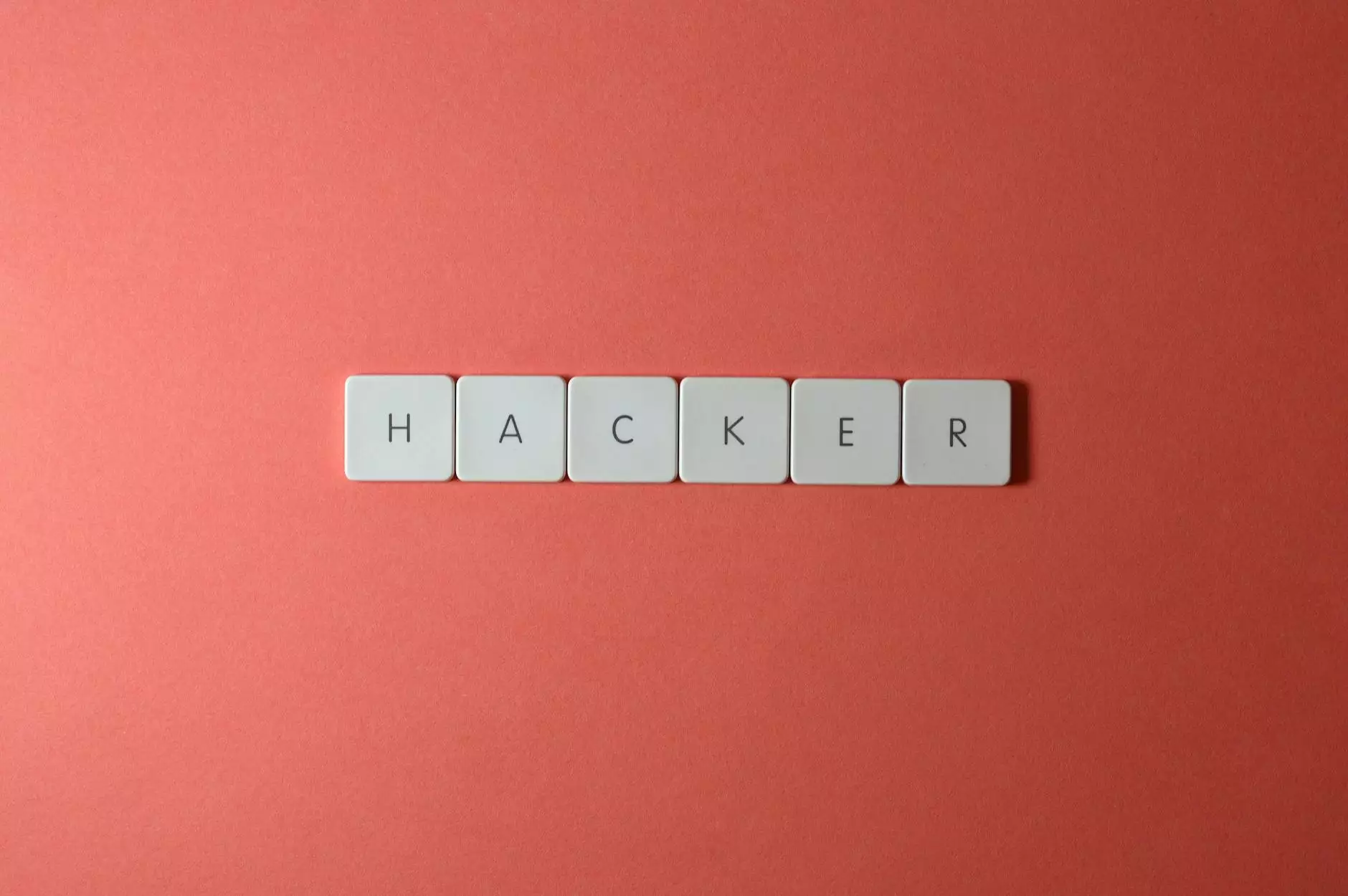
The digital landscape is continually evolving, bringing with it both unprecedented opportunities and significant challenges. One of the most pressing challenges faced by organizations today is the threat posed by malware. As cybercriminals become increasingly sophisticated, the need for effective malware detection solutions has never been more crucial. This is where machine learning in malware detection comes into play, ushering in a new era of cybersecurity.
Understanding Malware and Its Threats
Malware, short for malicious software, is a term that encompasses a range of harmful software programs designed to infiltrate, damage, or disable computers and networks. Common types of malware include:
- Viruses: Programs that attach themselves to clean files and spread throughout a computer system.
- Worms: Standalone software that replicates itself to spread to other computers.
- Trojan horses: Malicious software disguised as legitimate software.
- Ransomware: A type of malware that encrypts files and demands payment for their release.
- Spyware: Software that covertly collects user information without their consent.
As the methods employed by cybercriminals become more refined, it becomes increasingly difficult for traditional malware detection systems to keep pace. This is where machine learning comes into play.
The Role of Machine Learning in Malware Detection
Machine learning (ML) is a subset of artificial intelligence that enables systems to learn from data, identify patterns, and make decisions with minimal human intervention. When applied to malware detection, ML algorithms can analyze vast amounts of data, learning from previous attacks and adapting to new threats in real-time.
How Machine Learning Works in Malware Detection
The process of using machine learning for malware detection typically involves several crucial steps:
- Data Collection: Gathering a wide variety of data, including known malware samples, benign software, system logs, and user behavior patterns.
- Feature Extraction: Identifying the significant characteristics or features of the collected data that can help distinguish between malicious and benign software.
- Training the Model: Utilizing the processed data to train the machine learning model. The model learns to identify patterns associated with malware.
- Testing and Validation: Testing the model using a separate dataset to ensure accuracy and effectiveness at detecting new threats.
- Deployment: Implementing the trained model within the existing cybersecurity infrastructure to start detecting malware in real time.
Advantages of Machine Learning in Malware Detection
The integration of machine learning into malware detection systems offers several advantages:
- Improved Accuracy: Machine learning algorithms enhance detection rates by identifying previously unknown malware through behavior analysis rather than relying solely on predefined signatures.
- Real-time Detection: ML systems can analyze and respond to threats in real-time, reducing the window of vulnerability significantly.
- Adaptability: As new malware types emerge, ML systems can adapt their detection techniques based on the latest trends and patterns in the data.
- Reduced False Positives: Advanced algorithms minimize the number of false alarms, allowing IT teams to focus on genuine threats rather than sifting through non-issues.
- Cost Efficiency: Automation of threat detection helps lower the operational costs associated with manual malware analysis.
Challenges in Implementing Machine Learning for Malware Detection
While the benefits of using machine learning in malware detection are substantial, several challenges exist:
- Data Quality: The effectiveness of machine learning models largely depends on the quality and quantity of the data used for training.
- Complexity of Models: Developing and maintaining machine learning models require skilled personnel and can be resource-intensive.
- Adversarial Attacks: Cybercriminals can exploit the weaknesses in ML algorithms, crafting malware designed to evade detection by these systems.
- Integration Issues: Combining ML solutions with existing security frameworks can be challenging and may require significant modifications.
Case Studies of Machine Learning in Action
Several organizations have successfully implemented machine learning in their malware detection efforts, illustrating the capability and efficiency of this technology:
1. Darktrace
Darktrace has developed self-learning AI that monitors internal network activity to identify unusual patterns that could indicate a malware attack. Its ability to detect and respond to threats in real time showcases the power of machine learning in adjusting to the unique environment of an organization.
2. Cylance
Cylance uses machine learning algorithms to preemptively identify and neutralize malware before it can execute on a device. By analyzing code and behavior patterns, Cylance significantly reduces the risk of malware impacting system functionality.
The Future of Machine Learning in Malware Detection
The future of machine learning in malware detection appears promising. As technology continues to advance, several trends are expected to shape the landscape:
- Increased Automation: Automated systems will take on more roles in threat detection and response, freeing human analysts to focus on strategic decisions.
- Explainable AI: There will be a push for transparency in ML models, providing insights into how decisions are made and building trust in automated systems.
- Enhanced Collaboration: Organizations will share threat intelligence and collaborate using AI to improve collective defenses against malware.
- Greater Investment in AI Research: As the need for effective malware detection grows, so too will investment in research and development of machine learning technologies.
How Spambrella Enhances Machine Learning in Cybersecurity
At Spambrella, we recognize the critical importance of implementing cutting-edge technologies like machine learning in malware detection. Our team of IT professionals is dedicated to providing top-tier IT services and computer repair while utilizing advanced machine learning techniques to bolster our cybersecurity offerings.
Our approach includes:
- Customized Solutions: Tailoring machine learning algorithms to meet the specific needs of our clients based on their unique risk profiles.
- Continuous Monitoring: Providing ongoing threat detection and response services that leverage machine learning for real-time protection.
- Comprehensive Support: Ensuring our clients have access to expert advice and solutions tailored to their specific challenges in cybersecurity.
Conclusion: Embracing the Future of Cybersecurity
As the threat of malware continues to evolve, the necessity for advanced detection methods is undeniable. Implementing machine learning in malware detection not only enhances an organization’s ability to combat these threats but also fosters a proactive approach to cybersecurity. With partners like Spambrella, businesses can ensure they remain one step ahead in the fight against malicious software, protecting their assets and upholding the trust of their clients.
In a world where cybersecurity is paramount, leveraging innovative solutions such as machine learning is not just beneficial; it is essential for survival.